Overview
This is the official course webpage for ELE 530. Everything in blackboard just points here. Blackboard will only be used for some reporting of grades.
Announcements
- [2010/03/15] Final Exam on Wed., May 11 from 3-6pm in J323.
- [2010/02/28] Homework 3 has been posted.
- [2010/02/16] Homework 2 has been posted.
- [2010/02/02] Homework 1 has been posted.
- [2010/02/02] Welcome to ELE 530!
Course Information
Lectures
E-Quad J323
MW 3-4:20pm, 3 Units.
The lecture schedule shows what will likely be covered in each lecture this quarter.
Teaching Staff
- Instructor:
-
- Paul Cuff
- Office: B316 E-Quad
- E-mail: cuff@princeton.edu
- Phone: 609-258-7946
- Office Hours: MW 10-11:30am
Course Topics (pdf)
Fundamental Topics in Detection and Estimation:- Detection
- Hypothesis Test
- Neyman-Pearson Lemma
- Likelihood Ratio Test
- Kullback–Leibler Divergence
- Receiver-Operator Characteristics (ROC)
- Matched Filter
- Sequential Test
- Composite and Statistical Hypothesis Tests
- Estimation
- Minimum Variance Unbiased Estimators
- Cramer-Rao Bound
- Sufficient Statistics
- Maximum Likelihood
- Efficient estimators
- Bias
- Bayesian
- Minimum mean square error (MMSE)
- Linear MMSE
- Minimum absolute error
- Minimum probability of error (MAP)
- Methods to deal with complexity
- Expectation Maximization
- Graphical Models
- Hidden Markov Model
- Signals
- Stationarity and Power Spectral Density
- Wiener Filter
- Kalman Filter
Textbooks
- Main text:
- Additional text:
- Fundamentals of Statistical Signal Processing
Vol. 2 : Detection Theory
Steven M. Kay, Princeton Hall, 1998. - Linear Estimation
Kailath, Sayed, and Hassibi, Princeton Hall, 2000. - An Introduction to Signal Detection and Estimation
by H. Vincent Poor, 2nd Edition, Springer, 1998.
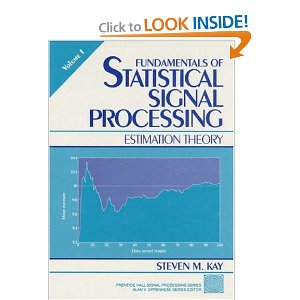
Fundamentals of Statistical Signal Processing
Vol. I : Estimation Theory
Steven M. Kay, Princeton Hall, 1993.
Prerequisites
- Probability
- Linear Algebra
- Signals and Systems
Exams
- Midterm:
- March 9 (Wednesday), 3-4:20pm
In class, open book - Final:
- May 11 (Wednesday), 3-6pm
In class, open book
Grading Policy
- Course Grade
-
The final grade received for the course will be based on homework, an optional project, and midterm and final exam scores. The grades are not combined as a weighted average as is usually done. Instead, we will use a soft-max (log-sum-exp) in the following formula:
Final grade = ln(exp(HW)+exp(project))
+ ln(exp(MT)+exp(Final)) + 1/2 Final.The homework and project are graded on a 5 point scale. The Midterm and Final will each be normalized to a mean of 5 and a standard deviation of 1. Based on the above formula, the final grades will be roughly on a 15 point scale. The log-sum-exp function returns a value greater than both arguments. You will benefit slightly (up to .693 points) by having high scores in both items rather than one.
Consequently, you must either do the homeworks thoroughly or complete an optional project. The higher of the two grades may contribute to around 40% of the course grade. You also have an opportunity to focus your effort and redeam yourself from a low score on the midterm if necessary. A high score on the midterm puts you in a good position, but you still need to learn the rest of the material and do well on the final.
Letter grades will be assigned monotonically from the final grade calculation.
- Homework Policy
-
There will be approximately 7 weekly homework assignments. These are not handed in. Instead, each student should make a 15 minute appointment during office hours to review the completed assignment in person. Please use the appointment scheduler to find a time that works for you. A student who chooses not to do the assignments may wish to use the time to discuss a project. The lowest homework scores will be dropped. Beyond that, exceptions will not be made for missed or late homework.
- Project
-
Students may optionally choose to do a project of their selection. Projects may involve researching the literature on current areas of interest in the field (and contributing to Wikipedia). Implementing the techniques discussed in this course through an applied project is also encouraged. The project grade will replace the homework grade, as explained above in the course grade section, yet the homework will still be important for mastering the material and performing well in the exams.
Time will be given for presentation of projects in class toward the end of the semester.
Handouts
Homework and Solutions
- Homework 1 (due Feb. 11)
- Homework 2 (due Feb. 25)
- Homework 3 (due March 7)
- Homework 4 (due April 4)
- Homework 5 (due April 13)
- Homework 6 (due April 27)
Handouts
- [2/02] #1: Course Topics (syllabus)
- [2/02] #2: Homework #1
- [2/16] #3: Homework #2
- [2/26] #4: Homework #1 Solutions
- [2/26] #5: Homework #2 Solutions
- [2/28] #6: Homework #3
- [3/08] #7: Homework #3 Solutions
- [3/28] #8: Homework #4
- [4/08] #9: Homework #4 Solutions
- [4/07] #10: Homework #5
- [4/21] #11: Homework #6
- [5/09] #12: Homework #6 Solutions