On Oct. 16-17, some 60 Princeton graduate students and postdocs — along with a handful of undergraduates — explored the most widely used deep learning techniques for computer vision tasks and delved into using new parallel computing programs to dramatically speed up applications.

Since an early flush of optimism in the 1950s, smaller subsets of artificial intelligence, first machine learning, then deep learning, have created ever larger disruptions.
The two daylong workshops were led by experts from the NVIDIA Deep Learning Institute and were sponsored by the Princeton Institute for Computational Science and Engineering (PICSciE), part of the Princeton Research Computing consortium and co-sponsored by the Center for Statistics and Machine Learning.
Deep learning, perhaps the most rapidly growing branch of machine learning, loosely models itself after the complex and non-linear way the human brain analyzes information and makes predictions. Computer vision programmers realized that, by the age of 3, young children have absorbed information about hundreds of millions of objects they see in the world around them; it’s this vast and varied input of data that trains them to recognize the same object in very different contexts.
“We train an artificial neural network to recognize and classify things in a somewhat similar way,” said NVIDIA solutions architect Brad Palmer. “The algorithm gets better at identifying something the more it sees that object in varied situations.” (A Princeton professor, Kai Li, the Paul M. Wythes and Marcia R. Wythes Professor in Computer Science and an associated faculty with PICSciE, was on the team that created the world’s largest visual database, Imagenet, a crucial training resource for computer vision programmers.)
One attendee of the deep learning workshop was Princeton computer science doctoral student Ksenia Sokolova, who works on a research project with Olga Troyanskaya, professor of computer science and the Lewis-Sigler Institute for Integrative Genomics and an associated faculty with PICSciE. Sokolova said she attended the workshop because of this technology’s potential in genomics research and precision medicine. “I am currently working on a deep learning model that would help us get better insight into the dependencies between mutations in the DNA and diseases the person might have,” she said.
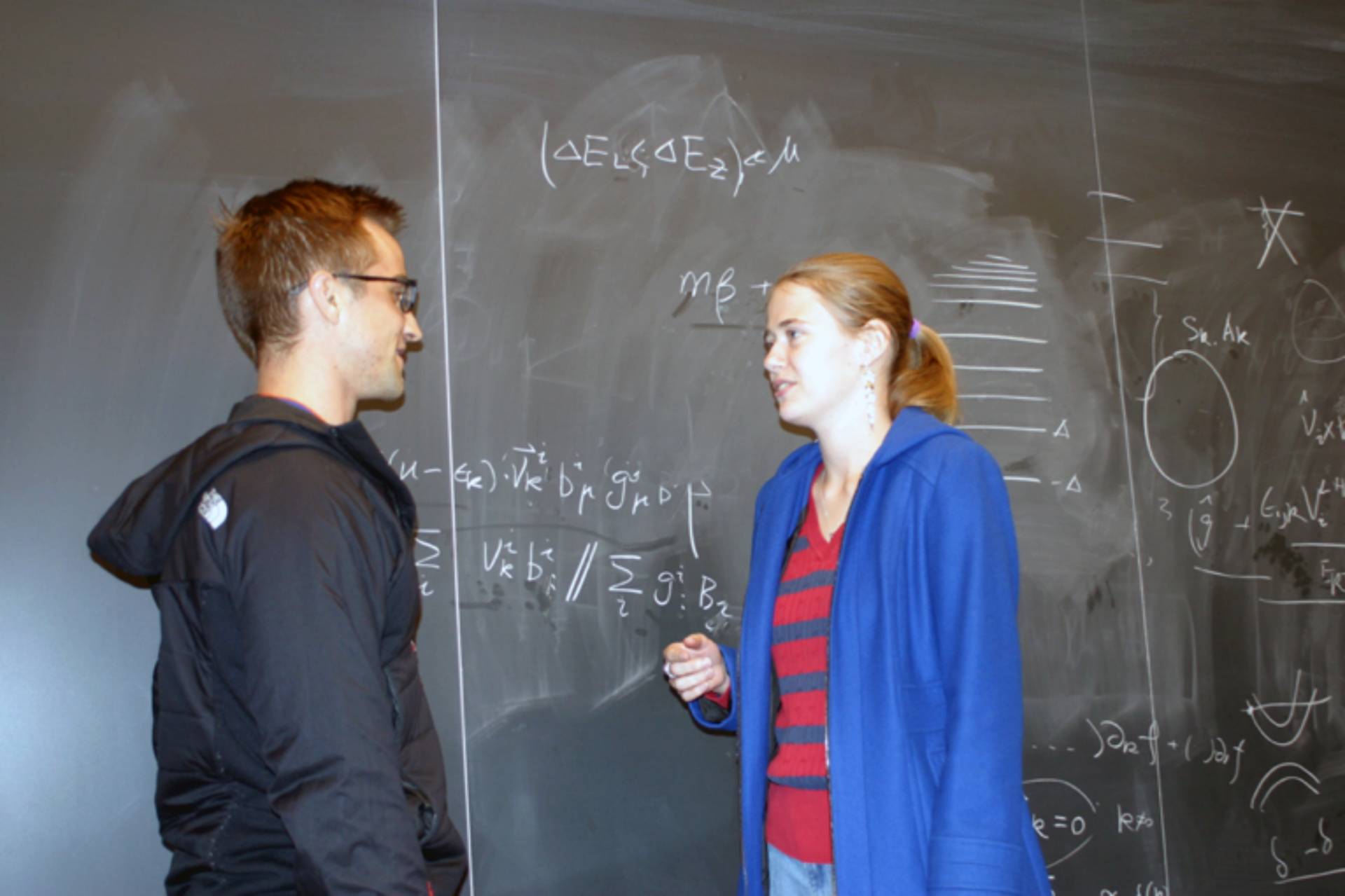
Computer science doctoral student Ksenia Sokolova, right, speaks with geosciences graduate student Ryan Manzuk during a break at the daylong Deep Learning workshop held in Jadwin Hall.
Added Sokolova: “Genomics is well-suited for deep learning; there are large amounts of complex data that needs to be interpreted. I think that it’s important to have a broad knowledge of these methods and applications, and I found this workshop to be a well-prepared and structured introduction into working with neural networks and their applications for computer vision.
“When people start to work in deep learning, they don’t realize the extent to which training parameters affect the performance. For example, it is possible low accuracy is due to the way the network is trained, and not because of the net architecture.”
“We can use that information to give a single impact score that indicates whether or not a mutation appears to be a human-disease mutation and whether it is likely to be functional,” Troyanskaya has said. Her cross-disciplinary team includes experts in bioinformatics, machine learning, statistics, algorithms and biology, working together to translate computational predictions into testable hypotheses about diverse areas of research such as Alzheimer's disease, autism, breast cancer and kidney disease.
Ronak Shah and Jonathan Bentz, two members of NVIDIA’s Higher Education Research team, noted that the “Princeton difference” is clear each time they interact with faculty and student researchers. “At Princeton we see a progressive approach to science with a uniquely high number of homegrown applications, such as the open-source software packages created by the Theoretical and Computational Seismology Group led by Jeroen Tromp,” said Shah. “I feel there is a strong correlation between Princeton’s high research output and the number of researchers developing new computational science techniques.”
Added Bentz, “The relationship between Princeton Research Computing and University research groups is really exciting to see. PICSciE and OIT Research Computing are organized in a way that results in a highly productive collaboration between the University’s high-performance computational resources, its staff and the research groups who continue to do ground-breaking work in their respective fields.”