
Abigail Doyle, the A. Barton Hepburn Professor of Chemistry, led a team of researchers from Princeton University and Merck who have developed state-of-the-art software to predict reaction yields while varying up to four components. Their software is designed to work for any reaction on any substrate, making it a powerful tool in expediting the synthesis of new medicines.
To manufacture medicines, chemists must find the right combinations of chemicals to make the necessary chemical structures. This is more complicated than it sounds, as typical chemical reactions employ several different components, and each chemical involved adds another dimension to the calculations.
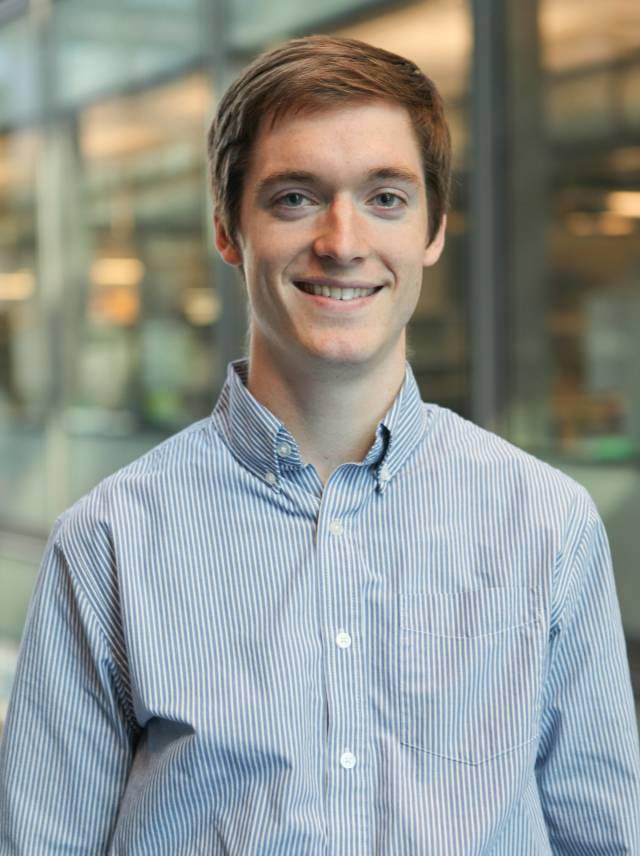
Derek Ahneman, a 2017 Ph.D. graduate, proposed using machine learning to model reaction yield while modifying four different reaction components, an exponentially more difficult endeavor than modifying one variable at a time. “At the outset, we knew there would be many challenges to overcome,” he said. “We weren't sure it was even possible.”
In an ideal world, chemists would like to predict which combination of chemicals would deliver the highest yield of product and avoid unintended by-products or other losses, but predicting the outcome of these multi-dimensional reactions has proven challenging.
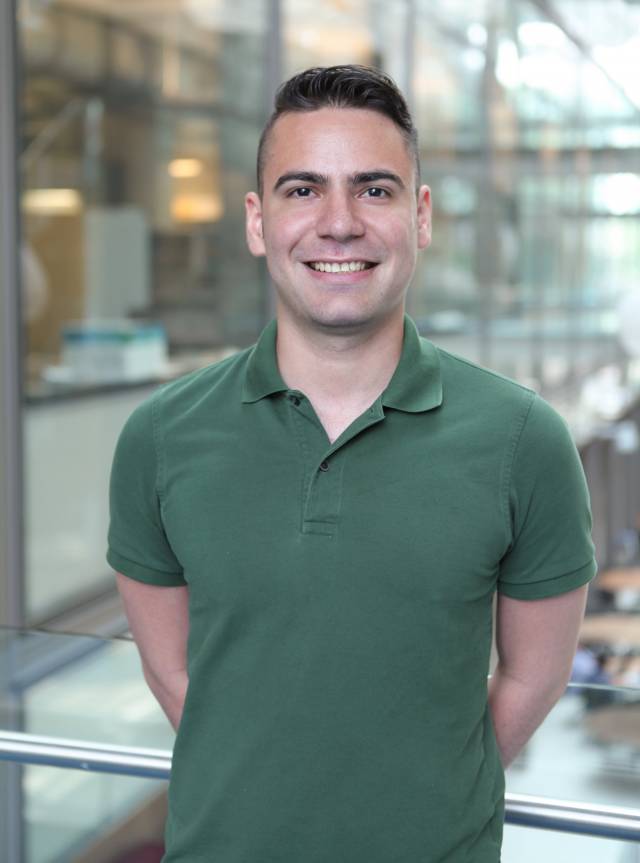
Jesús Estrada, a graduate student in Doyle's lab, finished developing the software that uses a "random forest" machine learning approach to predict chemical yields.
A group of researchers led by Abigail Doyle, the A. Barton Hepburn Professor of Chemistry at Princeton University, and Spencer Dreher of Merck Research Laboratories, has found a way to predict reaction yields accurately while varying up to four reaction components by using an application of artificial intelligence known as machine learning. They have turned their method into software that they have made available to other chemists. They published their research Feb. 15 in the journal Science.
“The software that we developed is designed to accommodate any reaction or substrate type,” said Doyle. “The idea was to let someone apply this tool and hopefully build on it with other reactions.”
Vast resources and time are expended to make synthetic molecules, often in a largely ad hoc manner, she said. Using this new software, chemists can identify high-yielding combinations of chemicals and substrates more cheaply and efficiently.
“We hope this will be a valuable tool in expediting the synthesis of new medicines,” said Derek Ahneman, who completed his chemistry Ph.D. in Doyle’s lab in 2017 and works for IBM.
“Many of these machine learning algorithms have been around for quite some time,” said Jesús Estrada, a graduate student in Doyle’s lab who contributed to the research and the paper. “However, within the synthetic organic chemistry community, we really haven't tapped into the exciting opportunities that machine learning offers.”
“As chemists, we’ve traditionally veered away from multi-dimensional analysis,” said Doyle. “We only look at one variable at a time, or a single set of conditions for a range of substrates.”
When Ahneman told Doyle that he wanted to use machine learning to tackle the multi-dimensional problem, she encouraged him. “I always — especially for my most talented students — try to give them free rein in the last year of their Ph.D.,” she said. “This is the project he proposed to me.”
Doyle and Ahneman set out to model reaction yield while modifying four reaction components, an exponentially more difficult endeavor than modifying one variable at a time.
“At the outset, we knew there would be many challenges to overcome,” Ahneman said. “We weren't sure it was even possible.”
Historically, one obstacle to developing multi-dimensional models has been collecting enough data on reaction yields to build an effective “training set,” he said. But recently, Merck has invented robotic systems that can run thousands of reactions on the order of days.
“I’m thrilled that the data we generated together was of very high quality, and they were able to create effective models,” said Dreher, a principal scientist with Merck's Chemistry Capabilities and Screening division. “Hopefully we can continue to develop this approach and can reduce our dependence on screening to make the designed molecules we need to make much faster.”
Another challenge has been calculating quantitative descriptors for each chemical to use as inputs for the model. These descriptors have typically been calculated one by one, which would have been impractical for the large number of chemical combinations they wanted to use.
They overcame this limitation by writing code that used an existing program, Spartan, to calculate and then extract descriptors for each chemical used in the model.
Once they had their quantitative descriptors, they tried several statistical approaches. First, they used linear regression, the industry standard, but found that it failed to accurately predict reaction yield. They then explored multiple common machine learning models and found that one called “random forest” delivered startlingly accurate yield predictions.
A random forest model works by randomly selecting small samples from the training data set and using that sample to build a decision tree. Each individual decision tree then predicts the yield for a given reaction, and then the result is averaged across the trees to generate an overall yield prediction.
Another breakthrough came when the researchers discovered that with random forests, “reaction yields can be accurately predicted using the results of ‘only’ hundreds of reactions (instead of thousands), a number that chemists without robots can perform themselves,” Ahneman said.
"Professor Doyle and her collaborators have applied artificial intelligence in a clever way to solve a problem that is poorly handled by simple linear models," said Julie Mitchell, a professor of mathematics and biochemistry at the University of Wisconsin-Madison who was not involved in the research. "In chemical space, small changes can have dramatic results, and such phenomena are better captured by their random forest model."
Doyle's team also found that random forest models can predict yields for chemical compounds not included in the training set.
“The techniques used are completely state-of-the-art,” said Chloé-Agathe Azencott, a machine learning researcher at the Centre for Computational Biology of Paris Science and Letters University, who was not involved in this research. “The correlation plots in the paper are good enough that I think we can envision relying on these predictions in the future, which will limit the need for costly laboratory experiments.”
“These results are exciting, because they suggest that this method can be used to predict the yield for reactions where the starting material has never been made, which would help minimize the consumption of chemicals that are time-consuming to make,” Ahneman said. “Overall, this methodology holds promise for (1) predicting the yield for reactions using as-yet-unmade starting materials and (2) predicting the optimal conditions for a reaction with a known starting material and product.”
After Ahneman finished his degree, Estrada continued the research. The goal was to create software that was accessible not only to computer experts like Ahneman and Estrada but the broader synthetic chemistry community, said Doyle.
She explained how the software works: “You draw out the structures — the starting materials, catalysts, bases — and the software will figure out shared descriptors between all of them. That’s your input. The outcome is the yields of the reactions. The machine learning matches all those descriptors to the yields, with the goal that you can put in any structure and it will tell you the outcome of the reaction.
“The idea is to help people navigate the multi-dimensional space where you can’t intuit the outcomes,” said Doyle.
The paper, “Predicting reaction performance in C–N cross-coupling using machine learning” by Derek Ahneman, Jesús Estrada, Shishi Lin, Spencer Dreher and Abigail Doyle, was published Feb. 15 in the journal Science. Financial support was provided by Princeton University, an Amgen Young Investigator Award and a Camille-Dreyfus Teacher Scholar Award.